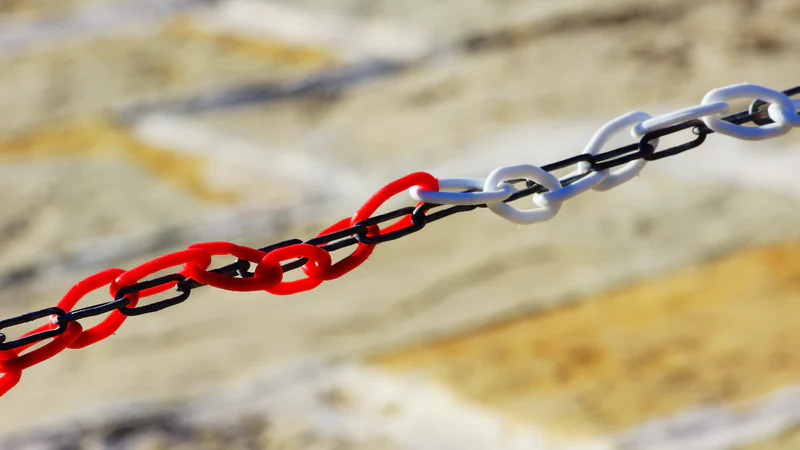
Since February, I along with my fellow classmates, have been embarking on a journey about machine learning and its operating system. This has also included practicing on a variety of problems.
Peter's confidence meter
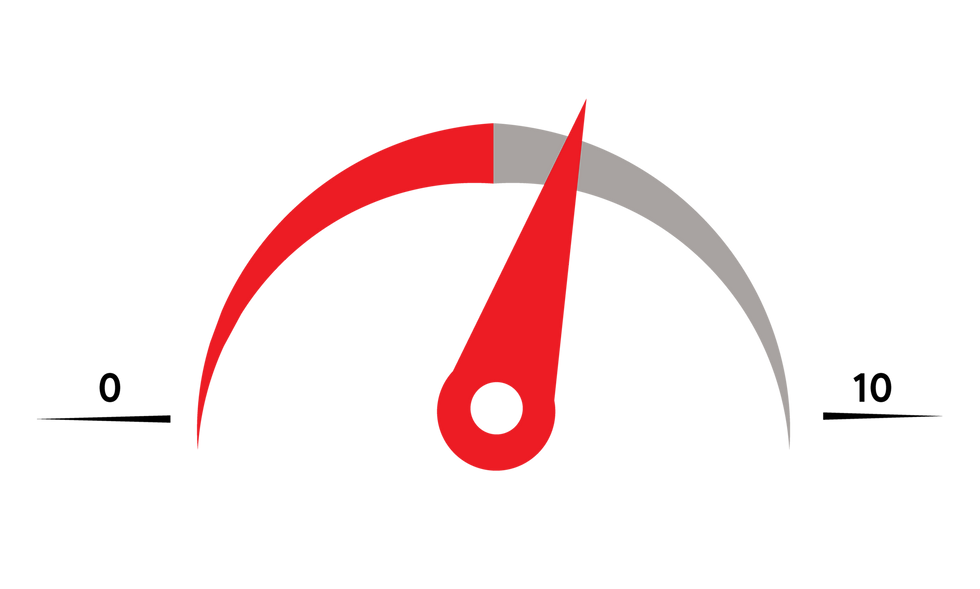
From my previous blogs, I can attest it has been a roller coaster ride with many highs and lows in understanding machine learning. In particular, understanding how we are going to apply machine learning in the real world.
Recently, the group had its last module together to recap the course and to discuss our on-the-job business project. Everyone had an opportunity to provide their thoughts and experience over the last several months, along with our level of confidence.
Some common themes that the group shared with everyone were:
Defining and understanding the problem is where much of your time should be spent.
Appling the machine learning process will guide you with your projects.
Solving the business problem is what you are trying to do – machine learning operating system plays a role.
Confidence level on average was five to six out of ten with most of my classmates. What struck me the most is with machine learning being so new, we all are building our confidence. One of our instructors Amit Varma, who owns an artificial intelligence company indicated that he continues to learn and evolve. He still seeks feedback from his colleagues or applies continuous improvements to his projects.
We are a community that will collaborate in sharing our successes when we solve challenges.
The highlight of the last module was the sharing of actual business cases where machine learning assisted in solving business challenges over the last year. With the pandemic in full force, the trucking industry was experiencing an increase in empty miles on return trips (2-3 times the industry average). This was putting a major strain on companies’ efficiencies and on profit margins. Machine learning was utilized to create a model that would predict which trips would come back empty allowing transportation companies to be upfront in charging a fair rate for the service.
The other case study was presented by Prabh Paul from Tracecost who shared a recent project where construction and supply chain management collaborated in utilizing machine learning to highlight potential project challenges in safe, materials and labor, hence reducing project cost errors. Over the next several weeks, I will be embarking on trying to classify our P-card spend to our enterprise taxonomy system. I look forward to the project as it brings together my learning with real case challenges.
Author - Peter Buscemi
Peter Buscemi brings energy in his role with Manitoba Hydro’s Supply Chain Management Strategic Sourcing group.
With over 25 years of business experience specializing in supply chain management, Peter has worked in a number of industries including Utilities, Public Sector, Retail, Distribution & Warehousing, Technology and Aerospace.
His past directorships include serving on Supply Chain Management Association’s (SCMA) Board of Directors along with local contributions with Winnipeg Chamber of Commerce and YES Winnipeg.
Peter holds a Supply Chain Management Professional (SCMP) designation and in 2015, he received the Fellow Award from the Supply Chain Management Association for his career achievements, contribution to the association and service to the community. It is the highest honour bestowed by SCMA.
Peter is actively involved in the community supporting the Kidney Foundation as a Board member. During his downtime, he enjoys cooking, gardening and time with his family.
Comentarios